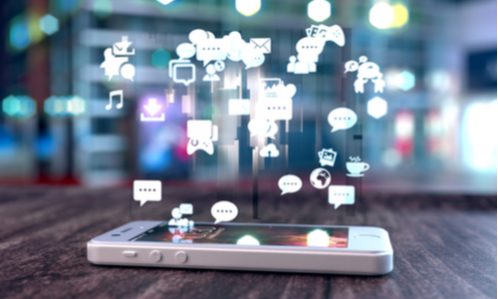
By: Juan Mateos-García & George Richardson (Nesta)
There’s a flurry of excitement about modern developments in artificial intelligence (AI).
The arrival of powerful image generators, AI agents able to perform multiple tasks or seemingly (to some) sentient chatbots are an exciting prospect for data scientists that use machine learning to tackle big societal challenges in areas such as health, education and the environment.
One of the unofficial remits of AI is to “solve intelligence and then solve everything else”. We have to assume that “solving” would include reducing inequalities in education, tackling obesity and decarbonising our homes. Are we about to get AI systems that could help us solve these problems?
Industrialised AI
The dominant model for AI is an industrial one. It trains deep, artificial networks on large volumes of web and social media data. These networks learn predictive patterns and can be useful for perception jobs such as identifying a face in a photo. They are good for tasks that don’t have human input or where we are not interested in understanding why someone made a choice, such as liking a social media post.
The large technology companies developing these systems use them to predict relevant search engine results, what social media content is most engaging and to make recommendations that could result in a purchase. This helps these companies build more engaging and profitable websites and apps.
But when it comes to social impact sectors, data is scarce, explanation is more important than prediction and making mistakes could cost lives.
An absence of data in the social sector
Our societies face big challenges: a widening outcome gap between the poorest children and the rest; growing obesity rates; the need to urgently reduce household emissions. AI systems such as those described above could help tackle these challenges by mapping the problems and targeting solutions. Unfortunately, this is easier said than done.
Search engines and social networking sites generate vast amounts of standardised data that is highly predictive of relevant outcomes. By contrast, social impact sectors such as education and health comprise hundreds or thousands of organisations (local authorities, hospitals or schools) each collecting small, incomplete and disconnected datasets. Social media or search engine data that could be relevant for improving outcomes in health (the food adverts different groups are exposed to, for example) or education (which social network structures increase community resilience and social mobility) are expensive or impossible to access. Even if it were possible, it’s likely they would provide a biased view of the situation, excluding or underrepresenting some vulnerable groups…
Featured News
UK Government Approves Vodafone-Hutchison Merger
May 9, 2024 by
CPI
Senate Majority Leader Announces Plan for AI Regulation Framework
May 9, 2024 by
CPI
BBVA Initiates Aggressive Takeover Bid for Sabadell
May 9, 2024 by
CPI
TikTok to Label AI-Generated Content Amid Election Interference Concerns
May 9, 2024 by
CPI
Italy’s Antitrust Authority Imposes Heavy Fines on Car Rental Giants
May 9, 2024 by
CPI
Antitrust Mix by CPI
Antitrust Chronicle® – Ecosystems
May 9, 2024 by
CPI
Mapping Antitrust onto Digital Ecosystems
May 9, 2024 by
CPI
Ecosystems and Competition Law: A Law and Political Economy Approach
May 9, 2024 by
CPI
Ecosystem Theories of Harm: What is Beyond the Buzzword?
May 9, 2024 by
CPI
Open Ecosystems: Benefits, Challenges, and Implications for Antitrust
May 9, 2024 by
CPI